HOW RAG ARCHITECTURES ARE TRANSFORMING GENERATIVE AI
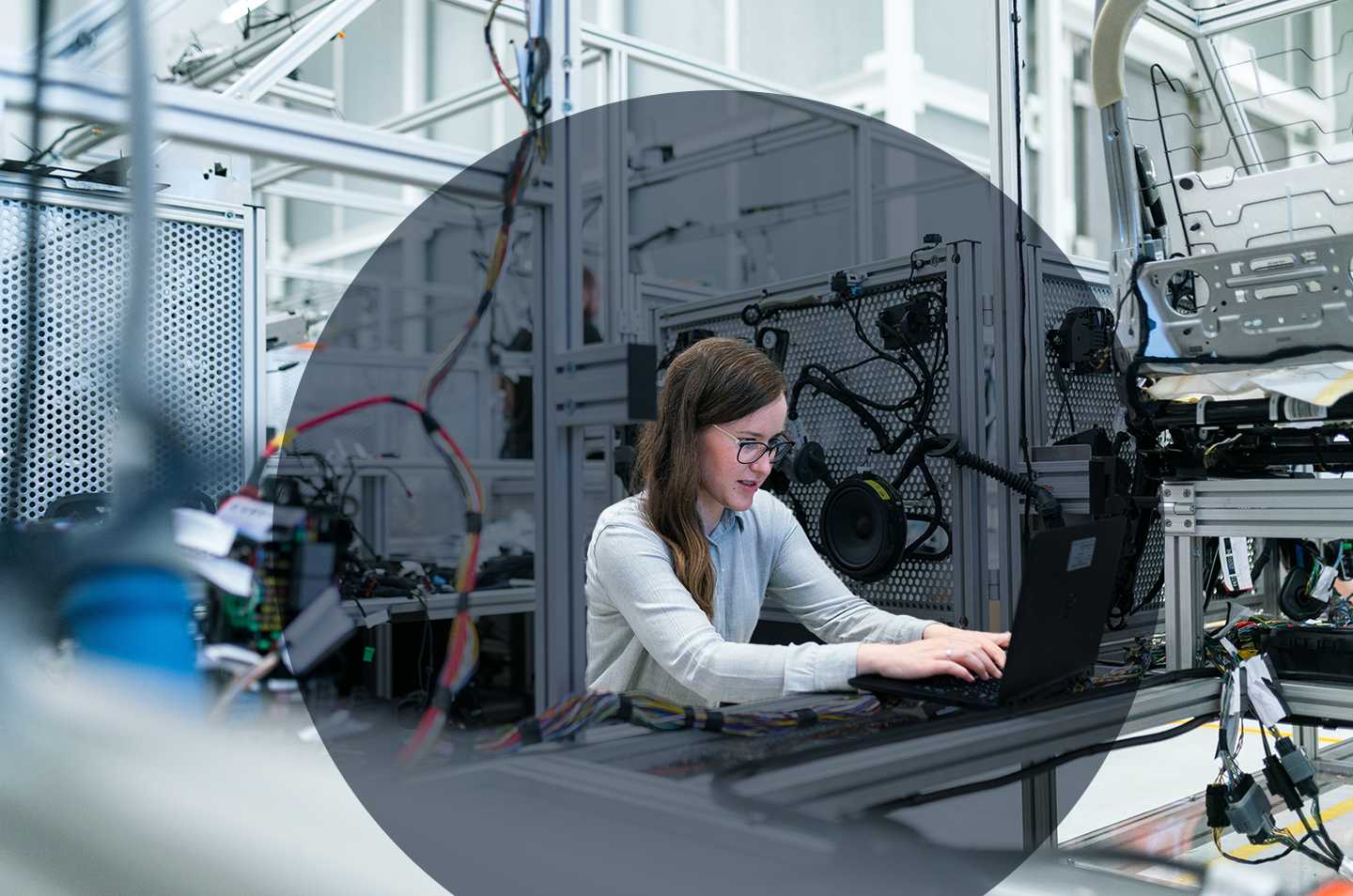
Although generative AI and large language models (LLMs) have been gaining widespread popularity, they have some inherent limitations.
LLMs are great at responding to general prompts because they are trained on enormous amounts of data to recognize patterns in human speech. Using vast amounts of data gives LLMs the ability to reason and generalize about many different tasks, but out of the box they lack deep domain-specific expertise. LLMs are inherently limited by their initial training datasets unless organizations undergo the time-consuming and costly process of fine-tuning or re-training the models using additional data.
Read on to learn how organizations can make the most of their LLM applications by taking advantage of the benefits of retrieval augmented generation.
What is RAG?
Retrieval augmented generation (RAG) is a way to improve the accuracy and reliability of LLMs by retrieving information from external sources. These external sources can be anything from industry-specific publications to proprietary company data. This transforms almost any LLM that was previously limited to insights derived from its training dataset into a dynamic generative AI system with access to domain-relevant data.
A RAG architecture retrieves information that’s relevant to a prompt as augmented context for the LLM. RAG differs from fine-tuning and re-training because external information is automatically added to the prompt rather than incorporating the additional knowledge into the model itself. This makes RAG an easier and more cost-effective way to give LLMs access to specialized or custom data.
The Benefits of RAG
One of the key barriers to the adoption of LLMs is user trust. Since LLMs rely on general patterns they’ve learned from their training datasets, they are prone to “hallucinating” or presenting incorrect information as factual. This erodes user trust and limits the efficacy of many generative AI solutions.
RAG helps overcome hallucinations by allowing LLMs to cite actual sources for the responses it gives. This means users can easily double-check any claims the LLMs provided by checking the sources. It also helps ground the AI model in verifiable information and establishes a transparent foundation for its responses. Plus, the ability to provide feedback on LLM responses is easily implemented in RAG and another way to make responses trustworthy.
Besides building user trust , RAG allows LLMs to have access to more relevant data. This could be more up-to-date information that goes beyond the cutoff date for its training dataset, which helps LLMs avoid outdated answers or hallucinating about something they haven’t been trained on. It’s usually faster and less expensive to implement RAG than it is to re-train a model with additional datasets.
How Organizations Can Leverage RAG
RAG is a powerful way for organizations to give any LLM access to current and domain-specific information without the costs of training or fine-tuning the model.
Here’s some ways enterprises are starting to leverage a RAG architecture:
- Customer Service: LLM-powered chatbots can leverage RAG to automatically deliver more useful answers based on company-specific data and knowledge bases. This can improve the customer experience by transforming generic chatbot responses into conversations that are highly relevant and contextually appropriate.
- Information Retrieval: RAG can augment LLM-powered search engines with internal data like company policies and data from enterprise systems, allowing employees to easily access company-wide knowledge repositories. This could also be governed by role, so finance teams can query data from financial systems and sales teams can query data from customer relationship management systems (CRMs).
The potential for RAG is huge, and many companies are starting to incorporate the architecture into their LLM applications. RAG is unlocking new possibilities for generative AI to deliver accurate and relevant responses that improve user experiences and drive better business outcomes.
With AHEAD’s extensive expertise in cloud and data engineering, our data science team has successfully engaged with multiple clients to execute RAG in a trustworthy, observable, scalable, and secure way for their organization.
Get in touch with us to learn more.